Instructor Led Training
Machine Learning with Python
Instructor-led training (ILT) is a traditional form of education that involves a skilled instructor leading a classroom or virtual session to deliver training to learners.
Limited seat available, enroll before date June 09, 2025.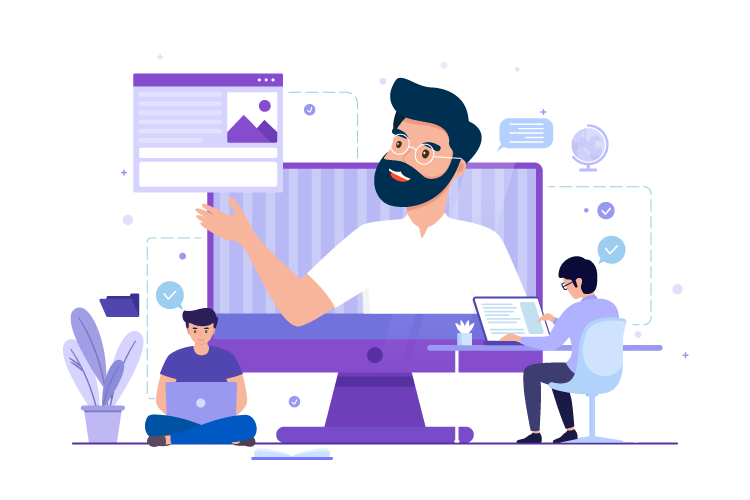
why should buy instructor led course?
Investing in an instructor-led course offers several advantages that can greatly enhance your learning experience. One of the key benefits is the opportunity to receive expert guidance from seasoned professionals who possess extensive knowledge and expertise in the subject matter. These instructors can offer valuable insights, address your queries, and provide guidance tailored to your specific needs. Additionally, instructor-led courses follow a well-structured curriculum, ensuring a comprehensive learning journey that covers all the essential topics. This structured approach enables you to progress in a logical and organized manner, building a strong foundation of knowledge. Moreover, instructor-led courses often provide personalized feedback, allowing you to receive individualized assessments and guidance to improve your understanding and skills.
Professional Certificate.
Obtaining certification of completion is a significant benefit that comes with many instructor-led courses. This certification serves as formal recognition of your successful completion of the course and showcases your commitment to learning and professional development. It can be a valuable addition to your resume or portfolio, highlighting your expertise and dedication in a specific field or skill set. Certification demonstrates to employers, clients, or colleagues that you have acquired the necessary knowledge and skills to perform tasks effectively. It can enhance your credibility and open doors to new career opportunities or advancements. Moreover, certification provides a sense of accomplishment and satisfaction, validating the time and effort you invested in the course. Ultimately, the certification of completion offers tangible evidence of your commitment to continuous learning and professional growth, making it a worthwhile asset in today's competitive job market.
How Does It Work?
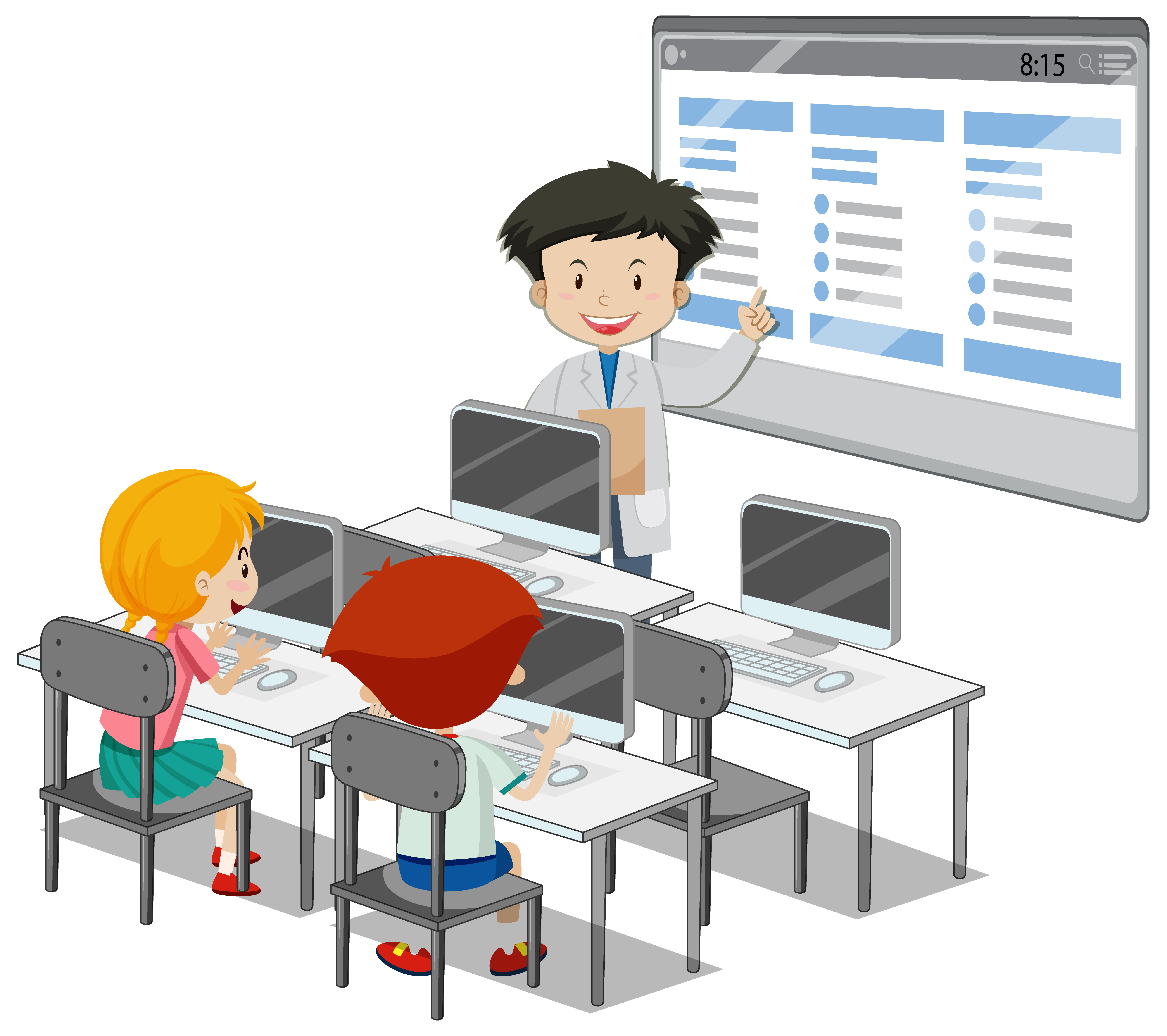
Zoom meeting with student twice a week.
As an educator, I have implemented a structured learning approach by conducting Zoom meetings with my students twice a week. This interactive platform has become an invaluable tool for fostering meaningful connections and facilitating engaging discussions in a virtual classroom setting.
AI Tutor support.
Mentoring support plays a crucial role in guiding individuals towards personal and professional growth. By offering mentorship, I provide a safe and supportive space for individuals to explore their goals, challenges, and aspirations.
Assignments and Grade.
Assignments and grading are essential components of the educational process, allowing students to demonstrate their understanding of concepts and skills while providing teachers with a means to assess their progress. Assignments are designed to reinforce learning, encourage critical thinking, and promote independent problem-solving.
About This Course
Learn Machine Learning with Python, a comprehensive training manual that teaches the fundamentals of coding with Python. Whether you want to improve your coding skills or you want an upgrade at your workplace, this is the ideal start. In this course, you’ll master the processes, patterns, and strategies of this user-friendly programming language. This Python ML course covers supervised learning paradigms, like classical algorithms, and regression techniques to evaluate performance metrics. Besides this, you’ll also learn feature engineering for converting raw data into meaningful features. Furthermore, you’ll also leverage the Python scikit-learn library along with other powerful tools. Practice on our Labs to solidify your understanding as you explore object-oriented programming, modules, error handling, and even file operations. By the end of this course, you'll be confidently writing Python ML scripts and resolving coding issues.
Skills You’ll Get
Lesson Plan
Let’s Discuss Learning
- Welcome
- Scope, Terminology, Prediction, and Data
- Putting the Machine in Machine Learning
- Examples of Learning Systems
- Evaluating Learning Systems
- A Process for Building Learning Systems
- Assumptions and Reality of Learning
- End-of-Lesson Material
Some Technical Background
- About Our Setup
- The Need for Mathematical Language
- Our Software for Tackling Machine Learning
- Probability
- Linear Combinations, Weighted Sums, and Dot Products
- A Geometric View: Points in Space
- Notation and the Plus-One Trick
- Getting Groovy, Breaking the Straight-Jacket, and Nonlinearity
- NumPy versus “All the Maths”
- Floating-Point Issues
- EOC
Predicting Categories: Getting Started with Classification
- Classification Tasks
- A Simple Classification Dataset
- Training and Testing: Don’t Teach to the Test
- Evaluation: Grading the Exam
- Simple Classifier #1: Nearest Neighbors, Long Distance Relationships, and Assumptions
- Simple Classifier #2: Naive Bayes, Probability, and Broken Promises
- Simplistic Evaluation of Classifiers
- EOC
Predicting Numerical Values: Getting Started with Regression
- A Simple Regression Dataset
- Nearest-Neighbors Regression and Summary Statistics
- Linear Regression and Errors
- Optimization: Picking the Best Answer
- Simple Evaluation and Comparison of Regressors
- EOC
Evaluating and Comparing Learners
- Evaluation and Why Less Is More
- Terminology for Learning Phases
- Major Tom, There’s Something Wrong: Overfitting and Underfitting
- From Errors to Costs
- (Re)Sampling: Making More from Less
- Break-It-Down: Deconstructing Error into Bias and Variance
- Graphical Evaluation and Comparison
- Comparing Learners with Cross-Validation
- EOC
Evaluating Classifiers
- Baseline Classifiers
- Beyond Accuracy: Metrics for Classification
- ROC Curves
- Another Take on Multiclass: One-versus-One
- Precision-Recall Curves
- Cumulative Response and Lift Curves
- More Sophisticated Evaluation of Classifiers: Take Two
- EOC
Evaluating Regressors
- Baseline Regressors
- Additional Measures for Regression
- Residual Plots
- A First Look at Standardization
- Evaluating Regressors in a More Sophisticated Way: Take Two
- EOC
More Classification Methods
- Revisiting Classification
- Decision Trees
- Support Vector Classifiers
- Logistic Regression
- Discriminant Analysis
- Assumptions, Biases, and Classifiers
- Comparison of Classifiers: Take Three
- EOC
More Regression Methods
- Linear Regression in the Penalty Box: Regularization
- Support Vector Regression
- Piecewise Constant Regression
- Regression Trees
- Comparison of Regressors: Take Three
- EOC
Manual Feature Engineering: Manipulating Data for Fun and Profit
- Feature Engineering Terminology and Motivation
- Feature Selection and Data Reduction: Taking out the Trash
- Feature Scaling
- Discretization
- Categorical Coding
- Relationships and Interactions
- Target Manipulations
- EOC
Tuning Hyperparameters and Pipelines
- Models, Parameters, Hyperparameters
- Tuning Hyperparameters
- Down the Recursive Rabbit Hole: Nested Cross-Validation
- Pipelines
- Pipelines and Tuning Together
- EOC
Combining Learners
- Ensembles
- Voting Ensembles
- Bagging and Random Forests
- Boosting
- Comparing the Tree-Ensemble Methods
- EOC
Models That Engineer Features for Us
- Feature Selection
- Feature Construction with Kernels
- Principal Components Analysis: An Unsupervised Technique
- EOC
Feature Engineering for Domains: Domain-Specific Learning
- Working with Text
- Clustering
- Working with Images
- EOC
Connections, Extensions, and Further Directions
- Optimization
- Linear Regression from Raw Materials
- Building Logistic Regression from Raw Materials
- SVM from Raw Materials
- Neural Networks
- Probabilistic Graphical Models
- EOC
Appendix A: mlwpy.py Listing
Frequently asked questions
Ready to get started?